AI can become an incredibly valuable tool for humanity, but it’s vital for stakeholders to work together now to ensure that the technology is safe, sustainable, and equitable. In this article, we will discuss some of the key concerns surrounding AI, and how startups, investors, and policymakers can address those concerns.
The Promise and Potential of AI
The past year has seen a boom in funding for AI startups. Even as funding and valuations recede in the startup ecosystem as a whole, AI is a sector which has seen continual funding as investors are excited by the rapid advancements in the quality of the technology.
Figure 1: Steady investment into AI Industry despite VC slowdown
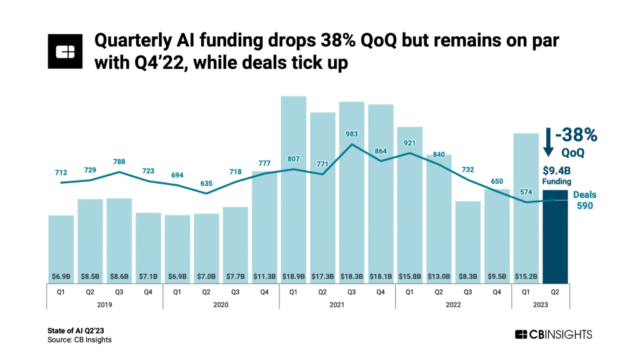
Source: CB Insights
It’s clear that AI has matured to a level where it can replicate or enhance many tasks which are currently being performed by humans. Already, institutions are leveraging AI to optimize everything from portfolio management to process automation, which can help boost performance and improve production costs. Since the launch of GPT-4 earlier this year, Generative AI has captured the public imagination and given us new tools for creativity and productivity in our personal and professional lives.
We can also see that tackling the world’s biggest issues such as financial inclusion and climate change will require the support of AI technology to not only accelerate the development of new solutions, but also to help deploy solutions at mass scale. Many experts have identified lack of data as a huge barrier for the transition to a sustainable economy – without reliable data and the ability to understand it, businesses may not know how or where to adjust their operations, and investors will struggle to deploy capital efficiently into the most impactful solutions. AI could be key solving these problems. Starting all the way at the first step of decarbonization a business, identifying and measuring the business’s GHG emissions, AI could be used to automate calculations by scraping and analyzing the organization’s existing information. This could emissions reporting feasible for SMEs who may lack the extra manpower or resources needed to manually evaluate their processes. Algorithms trained on satellite and climate data could help monitor and identify problem spots, and give governments better tools to prepare for natural disasters before they occur. Deloitte has identified other areas where AI could be used to drive sustainability, including “energy modelling for infrastructure optimization and urban planning; utilizing diverse data sources for environmental monitoring and targeted sustainability…; and the acceleration of climate science (physics emulators, climate forecasting, materials science).” [1]
From a social perspective, properly trained AI could revolutionize accessibility to healthcare and education. As will be discussed later in greater detail, use of AI in this space has several potential pitfalls, but there is still great opportunity for AI to serve the public good. One could imagine a world where chatbots and talkbots can take over many routine questions and screening in healthcare, freeing up valuable human resources to focus their efforts on patient care. AI algorithms have already been developed to assist doctors in analyzing medical data and diagnosing illnesses; speed and efficiency are important factors in reducing the cost of healthcare. Similarly, many startups are looking to use AI and technology to improve access to quality education, whether by providing tools that help teachers plan their curriculum and manage classrooms, or by developing applications to help students learn in non-classroom settings.
Despite the immense promise of AI technology, there remain many hidden or unrecognized costs related to the use of AI, from both a social and environmental perspective. Startups and investors alike need to be aware of the ESG risks associated with AI, and how those risks affect their future value generation.
Hidden Environmental Costs
From a sustainability perspective, the development of generative AI models in particular presents a problem. Generative AI models are trained on massive amounts of unstructured data, which requires massive amounts of computing power. As a result, training new models consumes high amounts of energy (to power the computers and servers) and water (used in cooling systems to ensure the computers continue to run at high capacity).
Researchers have estimated that training a single large-language model (LLM) could emit more than 626,000 pounds of carbon dioxide emissions (CO2e).[2] For reference, the average human is only responsible for roughly 11,000 tons of CO2e across a full year. According to the researchers’ analysis, the process of fine-tuning these types of large models is responsible for much of the estimated emissions, which presents an ethical quandary as fine-tuning is necessary for ensuring the accuracy and usability of an AI model, not to mention giving data scientists the opportunity to test and adjust the models to ensure the results are safe and equitable.
Figure 2: Carbon Emissions of Model Training, Relative to Benchmarks
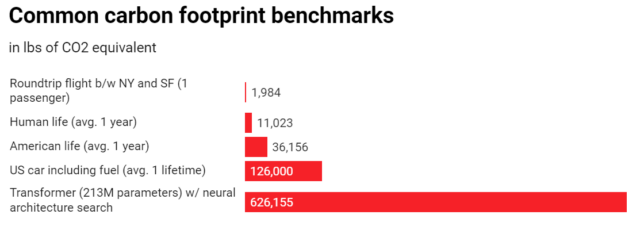
Source: MIT Technology Review, Strubell et. al.
While carbon emissions may be one of the more pressing concerns for investors and financial institutions (particularly with increasing regulatory pressure regarding emissions reporting and taxation), training AI models also has an effect on water consumptions and scarcity. Researchers have estimated that training AI models such as GPT-3 consumed roughly 700,000 liters of freshwater. Large tech giants such as Microsoft and Google have already begun paying increased attention to water consumption as a key environmental risk in their sustainability reports.
Social and Ethical Considerations
There have been numerous concerns raised regarding the further development and usage of AI technology. Some concerns are specific to AI, including problems related to data privacy, model biases, and algorithmic decision making. Other concerns are more philosophical in nature, such as the impact of AI on jobs and society. Such issues have been endemic to technological innovation throughout history, but given the immense changes in work and life that may result from AI, it remains vital for stakeholders to consider how best to face these challenges.
In the past few years as AI algorithms have been deployed at increasing large scale, multiple companies have run into problems when the technology has produced unforeseen results. For example, in 2016 Microsoft launched an experimental chatbot Tay; within 24 hours of launch, Tay had learned to produce profanity, racist comments, and controversial political statements.[3] More recently, a US lawyer was fined for using ChatGPT to prepare citations for a court filing when it was discovered that ChatGPT had fabricated some of the cases cited.[4] In a world where disinformation is already rampant throughout the internet, the emergence of generative AI models trained on massive amounts of data scraped from the internet raises concerns not only regarding accidental production of problematic content, but also intentionally malicious use of AI technology. Earlier this year, Check Point Research found that cybercriminals have begun experimenting with generative AI to more quickly develop malware or other cyber-attacks, despite their lack of programming skills.[5]
Use of AI in products such as digital lending, biometrics, and health diagnostics can also cause real harm to society if developers are not careful in screening for biases. Complex models like neural networks and deep learning are inherently a black box – it is difficult for people to understand how they make decisions. Black boxes are particularly susceptible to societal biases embedded in datasets used to train the model – research by ProPublica in 2016 revealed that machine learning-based risk assessments used in several jurisdictions for criminal sentencing were not only unreliable at predicting future crimes, but that the model’s errors were also racially biased. ProPublica’s analysis of the model’s performance found that black defendants were more likely to be misclassified as high risk, whereas white defendants were more likely to be misclassified as low risk.[6] In the field of health diagnostics, many datasets available to be used in training AI models contain primarily data on men, which creates inaccuracies when those same models are applied to predict illness or medical conditions for women.[7]
Apart from the problems with imperfect algorithms, many people are also concerned about the problems presented by the use of AI to replace human labor. This problem is not new, and has occurred over and over again throughout history with new technological developments. Economists consider “technological unemployment” as one of key types of structural unemployment. In this past, technological unemployment has been a temporary issue, as economies adapt and the labor force is able to reskill and retrain for new roles generated by advancements in technology. The fear however, is that advancements in AI and automation in this era may create a more permanent level of unemployment as companies are able to outsource an increasing number of tasks to computer algorithms. This concern is likely to disproportionately affect workers in emerging markets whose jobs may be the easiest to replicate using AI.[8]
Figure 3: Rapid acceleration of technological development
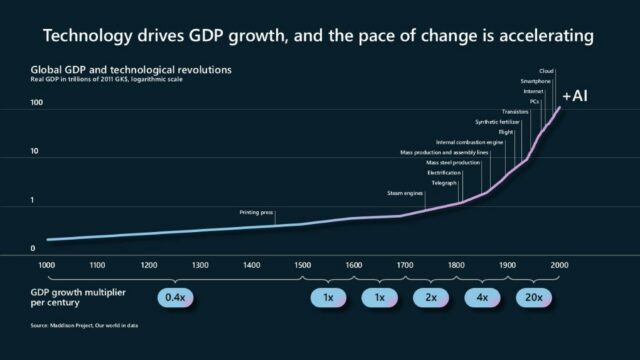
Source: https://blogs.microsoft.com/on-the-issues/2023/05/25/how-do-we-best-govern-ai/
What Can We Do About This?
There is no easy solution to any of these issues, but the potential of AI to create real positive impact on human life makes it vital for stakeholders to deeply consider how to balance the ethical and societal concerns as well as the negative environmental impact of AI development.
Many industry experts already espouse the principles of Responsible AI; tech giants like Microsoft and Google who are deeply involved in AI development and research provide guidance and tools for developers to embed these principles into their workflow. The Responsible AI Institute is a non-profit which supports organizations with tools to develop and identify safe and trustworthy AI products. Different organizations may adhere to different principles to ensure fairness, though similar concepts are found throughout the AI development industry.
Figure 4: PwC’s Responsible AI Framework
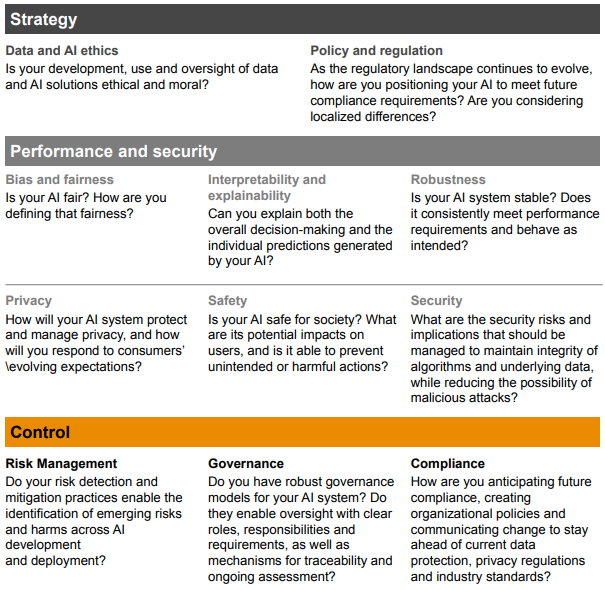
Source: PwC, Responsible AI – Maturing from theory to practice
Such frameworks may not be perfect (and the increasing complexity of AI algorithms makes them inherently prone to unexplainable errors), but they do represent a baseline of how startups can build trust and confidence with users and investors alike. Providing transparent documentation regarding data acquisition and sourcing, as well as model development and testing is key to ensuring that people do not feel like their privacy has been violated, and that they can fully trust the results produced by the system. Surveys by PwC also indicate that while many organizations are rapidly adopting such ethical principles in relation to their usage of AI technology, only 27% “are actively incorporating ethical principles in [their] day-to-day operations.”[9] Accordingly, many startups have seen this as a business opportunity, to create the tools necessary for other developers to improve their AI governance standards.
Figure 5: Responsible AI Startups
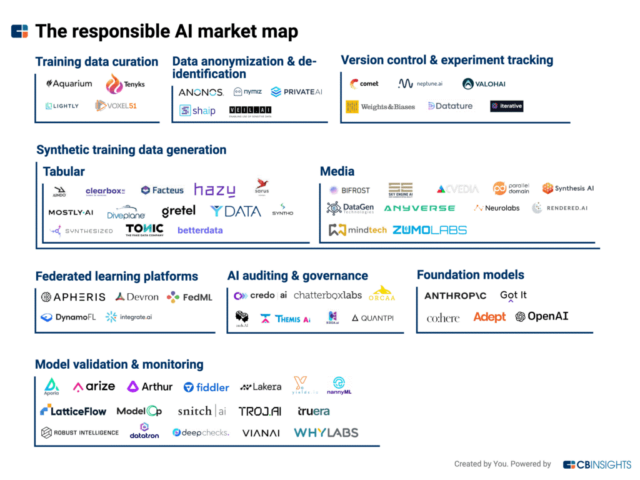
Source: CB Insights
Similarly, there are several recommendations for companies looking to decrease the emissions and resource consumption from their AI projects. These include:
- Leveraging and tuning existing models instead of training new models from scratch. Given the immense environmental cost associated with training new generative models, one of the simplest ways for companies to lower their environmental impact is simply to not train a new model, instead adapting pre-trained models for the company’s specific use case.
- Implementing efficient and energy-conserving computational methods. As energy usage is one of the key drivers of environmental impact, adopting low power computational approaches like tinyML[10] or efficient learning techniques like PARSEC[11] can help companies reduce both costs and energy consumption.
- Optimizing resource usage through selection of cloud providers and data centers. By shifting energy-intensive training to servers powered by clean energy, or which are located in places with more resource-efficient cooling systems, companies can lower their overall emissions and water usage. Depending on location, data centers may use significantly less freshwater, or may be linked to energy sources such as hydroelectric instead of fossil fuels.
The question of AI’s impact on human society may be both the most concerning issue and the issue which is hardest to find a solution to. Investors, policymakers, and startups alike need to consider the question of how we want AI to shape the future of human history, and how their actions will support the creation of AI products that are beneficial to humanity (as opposed to supporting the rise of Skynet). Many startups like Wiz.AI or Grammarly have developed AI tools that help human workers boost their productivity, as opposed to replacing human workers entirely. Ensuring that AI development is focused around augmenting human capabilities is one of the key ideas for mitigating some of the potential social downsides of technological development. This is also significant for ensuring the continued safety of AI development; one of Microsoft’s core principles for ethical AI development is to “always ensure that AI remains under human control.”[12]
For investors and financial institutions, it is important to embed these ideas into the due diligence process when considering whether to fund a new AI startup or project. Considering the value proposition of a project is already a key part of an investment decision, but in the context of AI investments, investors should be screening for whether the target is aware of the ESG risks and taking steps to mitigate those risks. Given the environmental costs (which may remain significant regardless of any mitigation plans), investors should also be considering whether the value proposition is sufficient to justify the costs. Regulators are already moving in favor of greater accountability surrounding ESG reporting and the reduction of financed emissions, thus it is the responsibility of investors to deeply consider how to balance these risks.
Finally, it is important to note that policymakers will need to play a large role in setting reliable standards and guardrails around the AI industry. It is clear that adopting many of the principles discussed above may require high effort and investment, particularly in the short run, and companies may be reluctant to do so without pressure from investors or regulators. By working together with industry experts, policymakers should be able to identify the best practices for safeguarding the future of the world, and to enforce those standards across the entire industry.
Conclusion
While some may consider the issues raised in this article as evidence of the danger that AI presents to human life and reason enough to put a halt to AI development, we continue to believe that we are not doomed to a dystopian future. There is immense potential for AI to benefit the future of humanity, to support us in figuring out new and innovative solutions for a more equitable and more sustainable world. Nevertheless, there are real costs associated with AI in the present day, and it is incumbent on startups, investors, and policymakers alike to ensure that we are aware of these costs and incorporate them into our decision making. Our actions today, and the degree to which we are willing to strive for responsible action, will dictate how the development of AI and human life plays out in the future. The recommendations discussed here for how different stakeholders should consider balancing ESG risk when developing new AI products are not exhaustive or set in stone, and we firmly believe that continued research, investment, and conversation surrounding these issues is necessary for ensuring the safety, sustainability, and equitability of AI technology.
Author: Krongkamol deLeon
Editor: Woraphot Kingkawkantong
Endnotes:
[1] https://www2.deloitte.com/uk/en/blog/experience-analytics/2020/green-ai-how-can-ai-solve-sustainability-challenges.html
[2] https://www.technologyreview.com/2019/06/06/239031/training-a-single-ai-model-can-emit-as-much-carbon-as-five-cars-in-their-lifetimes/
[3] https://www.bbc.com/news/technology-35890188
[4] https://www.unsw.edu.au/news/2023/06/this-us-lawyer-used-chatgpt-to-research-a-legal-brief-with-embar
[5] https://research.checkpoint.com/2023/opwnai-cybercriminals-starting-to-use-chatgpt/
[6] https://www.propublica.org/article/machine-bias-risk-assessments-in-criminal-sentencing
[7] https://www.forbes.com/sites/carmenniethammer/2020/03/02/ai-bias-could-put-womens-lives-at-riska-challenge-for-regulators/?sh=b060094534f2
[8] https://futurism.com/un-report-robots-will-replace-two-thirds-of-all-workers-in-the-developing-world
[9] PwC, Responsible AI – Maturing from theory to practice
[10] https://www.tinyml.org/
[11] Probabilistic Neural Architecture Search, https://hkaift.com/a-deep-dive-into-efficient-ai/
[12] https://blogs.microsoft.com/on-the-issues/2023/05/25/how-do-we-best-govern-ai/
Sources:
https://hbr.org/2023/07/how-to-make-generative-ai-greener#:~:text=AI%20(and%20software%20in%20general,75%25%20reduction%20in%20operational%20emissions.
https://blogs.microsoft.com/on-the-issues/2023/05/25/how-do-we-best-govern-ai/
https://earth.org/the-green-dilemma-can-ai-fulfil-its-potential-without-harming-the-environment/#:~:text=Behind%20the%20scenes%20of%20AI’s,gas%20emissions%2C%20aggravating%20climate%20change.
https://www.scientific-computing.com/analysis-opinion/true-cost-ai-innovation
https://www.technologyreview.com/2019/06/06/239031/training-a-single-ai-model-can-emit-as-much-carbon-as-five-cars-in-their-lifetimes/
https://www.ey.com/en_in/ai/how-generative-ai-can-build-an-organization-s-esg-roadmap
Strubell et. al., “Energy and Policy Considerations for Deep Learning in NLP.”
Li et. al., “Making AI Less Thirsty: Uncovering and Addressing the Secret Water Footprint of AI Models.”
OECD Digital Economy Papers, “Measuring the Environmental Impacts of Artificial Intelligence Compute and Applications: The AI Footprint.”